Editor’s Note: At the 2025 Global Developer Pioneer Conference, PsiBot stood out as the only Chinese embodied AI company invited to the event. For the first time, it systematically unveiled its industry-leading VLA (Vision-Language-Action) foundation model, showcasing capabilities in generalized manipulation and long-horizon task reasoning. At the event, PsiBot’s Founder and CEO, Dr. Viktor WANG, presented a deep dive into how the company leverages reinforcement learning to break through the “last-mile bottleneck” of embodied intelligence. Through multimodal penetration, zero-shot generalization, and sequential long-horizon skill chaining, PsiBot is delivering truly human-replacing, general-purpose intelligence solutions for real-world applications in manufacturing, services, and beyond.
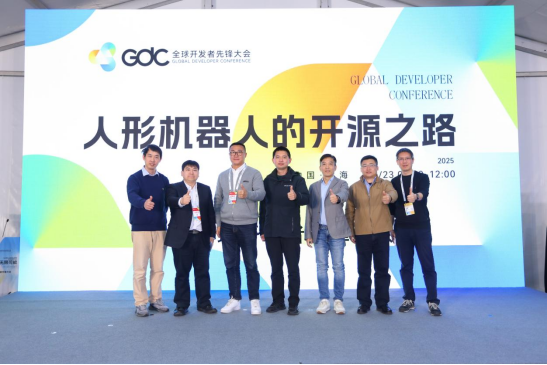
Psi-R0: The Industry’s First End-to-End Reinforcement Learning Embodied Model
Psi-R0: The Industry’s First End-to-End Reinforcement Learning Embodied Model
Psi-R0 is the first end-to-end embodied model released by PsiBot, built on reinforcement learning (RL). The model enables complex dual-handed manipulation, with multiple skills trained jointly in a chained manner to generate agents capable of reasoning and completing a full perception-to-action loop. Moreover, Psi-R0 demonstrates strong generalization across both objects and scenarios, setting a new standard for embodied intelligence.
DexGraspVLA: A Milestone in General-Purpose Dexterous Grasping Models
DexGraspVLA: A Milestone in General-Purpose Dexterous Grasping Models
DexGraspVLA achieved over 90% grasping success rate in a newly designed experimental environment featuring stacked scenes with over a thousand unseen object–lighting–background combinations. On the unseen single-object benchmark, it reached an impressive 98%+ success rate. Even under various environmental disturbances, the planner’s object recognition and labeling accuracy remained close to 100%, demonstrating remarkable robustness and generalization.
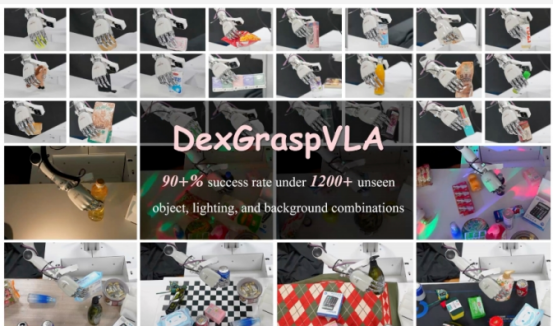
Align-DS-V: The Multimodal Version of DeepSeek
Align-DS-V: The Multimodal Version of DeepSeek
Align-DS-V is the first multimodal alignment framework built on the DeepSeek-671B trillion-parameter model. Designed to overcome the limitations of traditional AI in cross-modal reasoning, it enables deep integration of language, vision, and action modalities, delivering strong reasoning support for embodied intelligence applications.
Powered by slow thinking and strong reasoning, Align-DS-V continuously self-evolves beyond the constraints of single modalities. Through the deep fusion of world knowledge, it significantly expands the model’s intelligence boundaries—even within the text modality—laying the foundation for truly general-purpose multimodal cognition.
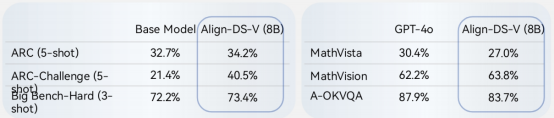
Align-DS-V Trial Access: https://ai.gitee.com/serverless-api?model=Align-DS-V
At the conference, Dr. Viktor WANG emphasized that there is still a significant journey ahead in enabling robots to understand the real physical world through models like DeepSeek. In current human-robot interaction, at least three core modalities are involved: language, vision, and force-based interaction (including more complex modalities such as tactile feedback).
While progress has been made from vision to language, achieving reasoning capabilities at the VLM (Vision-Language Model) level is still a work in progress. Generative models like Sora suffer from “memory loss” after just 60 seconds, and Google’s models begin to forget after 120 seconds—highlighting the limitations in sustained temporal reasoning across modalities.
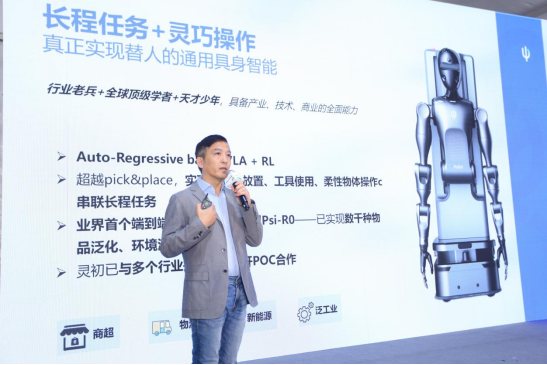
Dr. Viktor WANG also emphasized that DeepSeek-671B is an extremely large model, whereas Figure has shown in its Helix architecture that the System 2 “brain” module only needs to be around 7–8 billion parameters, while the “cerebellum” module can be as small as 80 million parameters—enabling the entire system to be deployed on the edge (on-device).
He pointed out that what’s most impressive about Figure is not just the scale of its model, but its real-time frequency performance: the brain module can run at 7–9 Hz, while the cerebellum can reach 200 Hz. In comparison, PsiBot’s current cerebellar inference runs at 30–50 Hz, while the brain typically operates at 1 Hz. As a result, PsiBot’s technical strategy is to perform training in the cloud, followed by lightweight model deployment on the edge for inference—a path the company firmly believes in.
Regarding the development and deployment stages of humanoid robots, Dr. Wang stated that embodied AI will continue to follow the pretraining paradigm of large models. These models will carry broad generalization capabilities for object understanding and skill learning. However, for real-world downstream tasks, significant post-training and fine-tuning are still required.
Today, PsiBot has gone beyond pretraining by taking two additional steps:
· It has developed its own exoskeleton devices to collect 1:1 real-world physical data,
· It applies that data for SFT-style fine-tuning, followed by real-world reinforcement learning to further optimize performance.
Conclusion: PsiBot is redefining the boundaries of embodied intelligence with a dual-engine approach driven by technical uniqueness and scenario-first execution. From foundational algorithms to real-world deployment, PsiBot offers a complete technology loop that provides developers with reusable infrastructure and injects new momentum into the intelligent transformation of manufacturing and services. As a key player in the general artificial intelligence race, PsiBot is accelerating the arrival of the “machine-as-human” era.