PsiBot has officially released its first end-to-end embodied model powered by reinforcement learning—Psi R0. This groundbreaking model enables complex dual-handed manipulation through skill-chained joint training, generating agents with reasoning capabilities that can autonomously complete and close the loop on long-horizon dexterous tasks. Psi R0 also supports cross-object and cross-scenario generalization at scale.
In the real world, nearly all human activities involve actions like grasping, rotating, pinching, and touching. Over 90% of these are multi-skill, long-horizon tasks. Yet in today’s embodied AI field, most models are still limited to generalizing simple Pick and Place operations. As tasks grow more complex and long-horizon, both generalization and success rates tend to collapse—making it difficult to deploy embodied systems beyond demo settings.
This fundamental limitation—robots stuck in short, rigid tasks and reliant on teleoperation—is a major barrier to real-world adoption. The key challenge is how to move beyond Pick and Place and empower robots with the ability to autonomously execute long-horizon, human-like manipulation in real, dynamic environments. Psi R0 is PsiBot’s bold first step toward solving this core problem.
Reinforcement Learning (RL) is the only viable solution for achieving task-level closure in long-horizon dexterous manipulation
In the real world, solving long-horizon tasks with robots requires a learning-based approach. Currently, there are two mainstream paradigms: Imitation Learning (IL) and Reinforcement Learning (RL).
Pure imitation learning suffers from limited generalization, constrained by the diversity and quality of demonstration data. Long-horizon tasks involve many sequential steps, which amplifies distribution shift issues, making IL less effective at generalizing and less robust in complex scenarios.
In contrast, PsiBot’s RL-based model, Psi R0, efficiently trains bimanual agents using large-scale simulated data and employs a bidirectional training framework to chain multiple skills. Psi R0 is the first in the industry to complete long-horizon tasks in open environments with strong generalization and high robustness.
This skill training framework abstracts key information from the spatiotemporal trajectories of objects to construct a general-purpose objective function, addressing the common challenge of designing explicit reward functions. During the post-training phase, alignment with a small amount of high-quality real-world data further improves the success rate in long-horizon tasks.
Additionally, the transition feasibility function within the bidirectional training framework plays a critical role. It fine-tunes each skill to improve overall success and generalization when executed in sequence. It also empowers the model with the ability to autonomously switch between skills—allowing it to rapidly adjust strategies in the face of failure and maintain high task success rates.

(Sequential Dexterity: Chaining Dexterous Policies for Long-Horizon ManipulationYuanpei Chen, Chen Wang*, Li Fei-Fei, C. Karen Liu)
The Psi R0 model demonstrates exceptional dexterity, high success rates, and strong generalization—highlighting its “brain” for task decomposition and planning, and its “cerebellum” for fine manipulation, generalization, and robustness. Its emergence marks a major breakthrough in overcoming the core technical bottlenecks that have hindered the commercialization of embodied robots. By unlocking new capabilities, Psi R0 paves the way for a vast new frontier in the field and is poised to lead embodied robotics into an entirely new phase of development.
From theoretical concept to practical deployment, Psi R0 delivers a definitive answer to the ultimate question of embodied AI commercialization.
Long-horizon dexterous manipulation tasks are ubiquitous—from assembly on factory lines, to picking and packing in the service industry, to tidying and cleaning in home environments.
Psi R0 showcases exceptional real-world applicability across these scenarios. In the context of e-commerce, for example, product packaging is a classic long-horizon task that involves grasping, scanning, placing, and tying plastic bags across tens of thousands of SKUs. Psi R0’s bimanual agent can perform this sequence of actions fluidly, effectively replacing an entire workstation on-site. It is the first embodied robot trained via reinforcement learning to complete such long-horizon dexterous operations in a production setting.
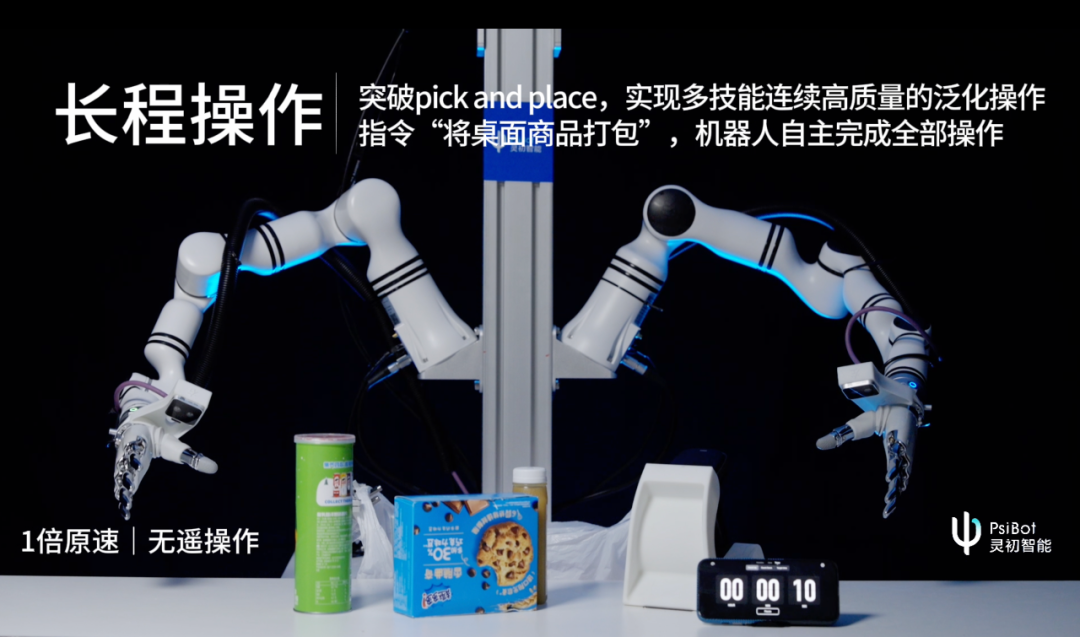
The only instruction received by the robotic system is simply: “Pack the items on the table.” Behind this seemingly straightforward command lies PsiBot’s highly innovative end-to-end architecture. Once the instruction is issued, an upper-layer Vision-Language Model (VLM) analyzes the disorganized assortment of items on the table and determines an execution order. The lower-layer manipulation model then decomposes the task for each individual item—such as grasping, placing, scanning, and bagging—executed sequentially by the agent.
In the grasping stage, the model must demonstrate strong generalization to handle objects of varying shapes and orientations placed arbitrarily. As shown in the video, Psi R0 was able to achieve a 99%+ success rate in grasping a can of Pringles using only 20 real-world data samples—a striking testament to its efficiency and generalization capability.
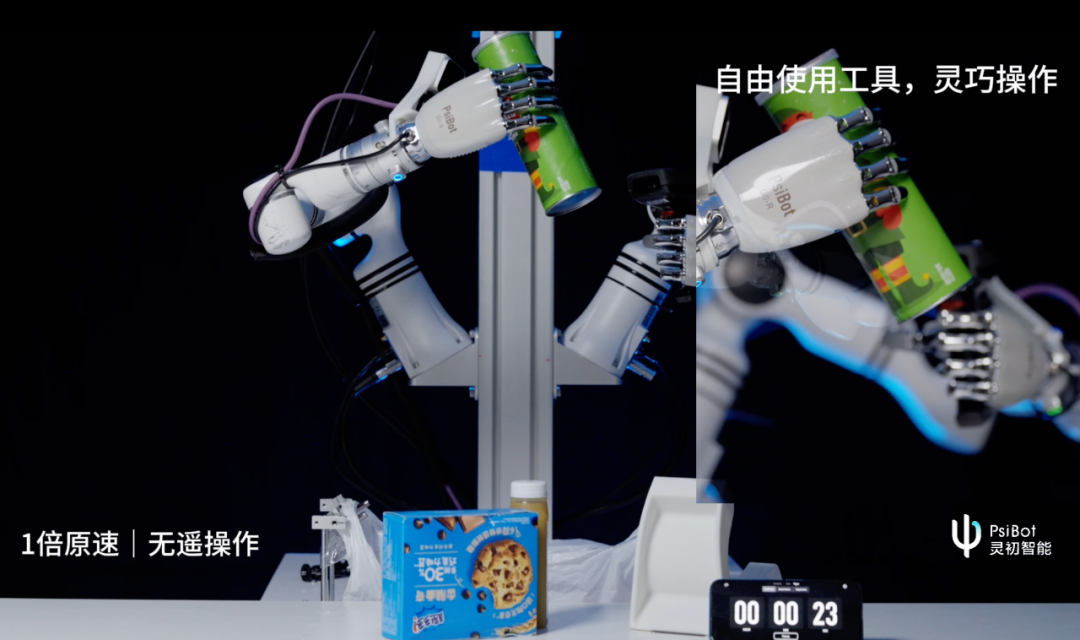
The scanning phase presents an even greater challenge for robotic dexterity, requiring both hands to coordinate with high precision to align the barcode scanner with the product’s barcode. Even the slightest misalignment can lead to failure. Here, reinforcement learning provides robust real-time closed-loop control for the high-DOF dual-arm system, enabling the scanning motion to be executed smoothly and accurately.
In the packaging phase, both hands must work together to manipulate a plastic bag with finesse. As the bag dynamically changes shape during the packing process, the robot must continuously adapt its manipulation in real time. To enhance the robot’s adaptability to deformable objects, Psi R0 simulates a wide range of flexible object scenarios in training and refines its performance with real-world data. Even when interrupted or disturbed, Psi R0 can autonomously adjust its strategy and reinitiate the packaging process—demonstrating exceptional robustness and adaptability in real-world settings.
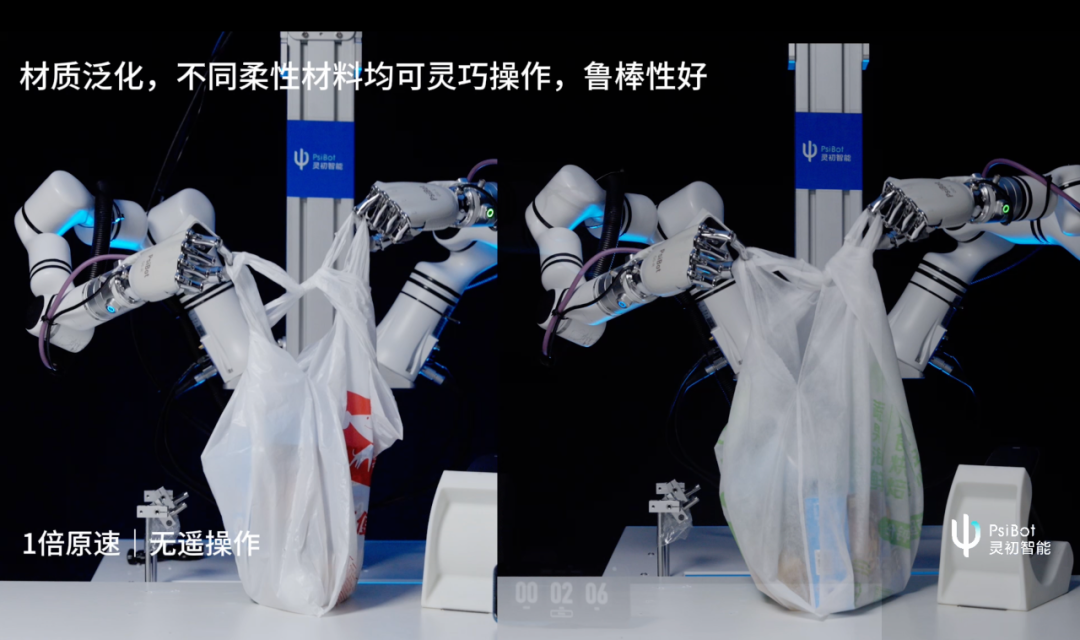
PsiBot’s Psi R0 model marks the first step in the recursive growth of embodied intelligence. Embodied AI follows a progressive evolution—from simple to complex, and from protective execution to collaborative autonomy.
In the early stages, the “cerebellum” serves as the physical foundation for real-world interaction. Its design must incorporate domain-specific knowledge to meet environmental constraints while maintaining fault tolerance to support learning and optimization by the “brain.” Psi R0 leverages the exploratory power of reinforcement learning to accelerate cerebellar iteration and generate agents capable of long-horizon dexterous manipulation.
By driving the data flywheel through dexterous action, Psi R0 establishes a closed feedback loop from action to cognition, enabling continuous optimization of cognitive capabilities. This forms a neural circuit of embodied intelligence—where the cerebellum supports and the brain evolves—allowing the end-to-end model to progress gradually from simple to complex behavior, and from safe execution to full-scale collaboration.